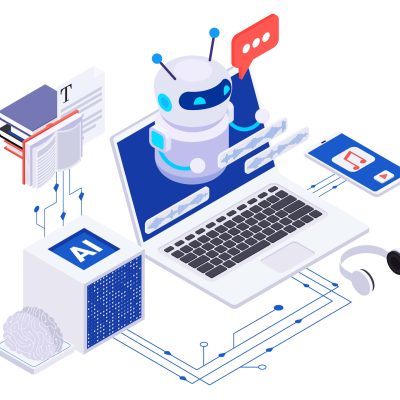
Artificial Intelligence (AI) has evolved rapidly over the years, transcending its traditional roles and transforming into a technology capable of creativity and imagination. One of the most fascinating developments in the field of AI is Generative AI, a subset that empowers machines to generate content that is remarkably human-like. From generating artwork and music to crafting compelling stories, Generative AI is revolutionizing industries and reshaping our perception of what machines can achieve.
Understanding Generative AI
Generative AI refers to a class of AI models that possess the remarkable ability to create new content, imitating human-like creativity. Unlike conventional AI, which typically involves rule-based programming or pattern recognition, Generative AI relies on neural networks and complex algorithms to learn patterns from existing data and then generate new, original content based on those patterns. This creative potential sets Generative AI apart, making it a powerful tool for innovation.
At the core of Generative AI are neural networks, particularly Generative Adversarial Networks (GANs) and Variational Autoencoders (VAEs). GANs consist of two parts: a generator and a discriminator. The generator crafts content, such as images or text, while the discriminator evaluates the content’s authenticity. Through a continuous feedback loop, the generator learns to produce increasingly realistic content. VAEs, on the other hand, focus on learning the underlying structure of data, enabling them to generate similar but distinct content.
Types of Generative AI
Generative AI comes in various flavors, each specializing in generating specific types of content:
1. Text Generation Models
Prominent examples like OpenAI’s GPT-3 and GPT-4 have gained widespread attention for their ability to generate coherent and contextually relevant text. These models can write essays, articles, stories, and even mimic the writing style of famous authors.
2. Image Generation Models
Models like DALL-E can generate images from textual descriptions, resulting in visually stunning and imaginative artwork. VQ-VAE-2 takes a different approach by encoding images into discrete codes, enabling it to generate high-quality images with remarkable diversity.
3. Music and Sound Generation Models
Generative AI is also delving into the realm of music composition. Models like MuseNet can create original compositions in various styles, and AI-driven tools are being developed to assist musicians in composing harmonious melodies.
Working Principles of Generative AI
The magic of Generative AI lies in its ability to learn from data. These models are trained on vast amounts of existing content, such as text, images, or music, which serve as the foundation for creativity. During the training process, the models learn the intricate patterns, styles, and nuances present in the data. Once trained, they can generate new content by extrapolating from the learned patterns.
Fine-tuning is a critical step in the process. Models are specialized for specific tasks or domains through additional training with focused data. For example, a language model can be fine-tuned to generate poetry by training it on a dataset of poems. This process enhances the model’s ability to generate content that aligns with the desired outcome.
Applications of Generative AI
Generative AI’s transformative potential is evident across diverse industries:
1. Art and Design
Generative AI is pushing the boundaries of artistic creation. DALL-E, for instance, can generate surreal and imaginative images based on textual prompts. Artists can collaborate with AI to produce unique pieces that blend human creativity with machine imagination.
2. Content Creation
Writers and content creators are finding companionship in AI models like GPT-3. These models can assist in generating articles, blog posts, and scripts, streamlining the content creation process.
3. Healthcare
In the realm of healthcare, Generative AI plays a role in medical image generation and analysis. It can create synthetic medical images to augment training data, aiding in the development of more accurate diagnostic tools.
4. Gaming
Generative AI is revolutionizing game development. It can autonomously design characters, environments, and even entire narratives, providing game designers with a wealth of creative possibilities.
5. Fashion
Fashion designers are leveraging Generative AI to design innovative clothing and accessories. This technology can generate unique patterns, styles, and designs, fostering novel expressions in the fashion industry.
Ethical Considerations
As with any transformative technology, Generative AI raises ethical concerns:
1. Misuse and Fake Content Generation
The same technology that generates awe-inspiring art and useful content can also be misused to create fake news, misleading images, and malicious content, leading to potential misinformation and harm.
2. Intellectual Property and Copyright
The ownership of AI-generated content raises questions about copyright and intellectual property rights. Determining authorship and ownership becomes complex when machines are involved in the creative process.
3. Bias and Ethical Implications
Generative AI models learn from existing data, which can inadvertently perpetuate biases present in the data. Developers must be vigilant to ensure that AI-generated content is free from discriminatory or biased undertones.
Challenges and Future Directions
While Generative AI holds immense promise, it faces several challenges:
1. Realism and Consistency
Generating content that is consistently coherent and indistinguishable from human-created content remains a challenge. Striking the balance between creativity and coherence is an ongoing pursuit.
2. Data Quality and Quantity
The quality and quantity of training data significantly impact the output of Generative AI models. Ensuring diverse, representative, and reliable data is crucial for the success of these models.
3. Ethical Frameworks
Developing ethical guidelines and frameworks for Generative AI is essential to prevent misuse and bias. The AI community must collectively address these concerns to ensure responsible development and deployment.
The Future of Generative AI
The journey of Generative AI is just beginning. As research continues and technology advances, we can anticipate even more remarkable achievements:
1. Personalization and Creativity Enhancement
Generative AI could be integrated into creative processes to assist artists, writers, and designers, offering suggestions and expanding their creative horizons.
2. Cross-Domain Creativity
Future models might possess the ability to generate content across different domains. Imagine a model that generates an entire multimedia presentation, incorporating text, images, and music seamlessly.
3. Collaborative Creation
Human-AI collaboration could become the norm. Artists, writers, and other creators could work alongside AI models to jointly produce content that transcends individual capabilities.
Conclusion
Generative AI has emerged as a beacon of creativity in the realm of artificial intelligence. Its ability to generate art, music, and content that resonates with human sensibilities is awe-inspiring. While challenges and ethical considerations persist, the potential for positive impact across industries is undeniable. As we stand on the cusp of a new era of AI-assisted creativity, it is imperative to approach Generative AI with responsibility and an eye toward unlocking its full potential.